Integrating AI into Enterprise Architecture (EA) is no longer a futuristic concept—it is necessary for businesses seeking to enhance efficiency, drive innovation, and maintain a competitive edge.
AI can optimize workflows, improve decision-making, and enhance business agility. However, without a structured approach, AI adoption can create operational silos and introduce unnecessary complexity.
This article explores how organizations can integrate AI into their enterprise architecture, ensuring seamless adoption and maximizing value.
Understanding Enterprise Architecture and AI Integration
Enterprise Architecture (EA) is the strategic framework that aligns an organization’s IT infrastructure with its business objectives. Traditionally, EA has been structured around data management, application architecture, and IT governance. AI disrupts this framework by introducing intelligent automation, predictive analytics, and data-driven decision-making.
Seamlessly integrating AI into EA requires a redefined approach that ensures compatibility, scalability, and alignment with business goals.
Strategies for Seamless AI Integration
1. Develop a Comprehensive AI Strategy
Before integrating AI into EA, organizations must develop a structured AI strategy that aligns with their overall business objectives.
- Define Clear Objectives: Identify specific goals AI should achieve, such as automating workflows, improving customer experience, or enhancing operational efficiency.
- Identify Key AI Use Cases: Determine where AI can provide the most value. Common applications include fraud detection, supply chain optimization, and customer service chatbots.
- Engage Stakeholders: AI adoption should be a collaborative effort involving IT, business leaders, and data scientists. This ensures alignment between AI initiatives and business needs.
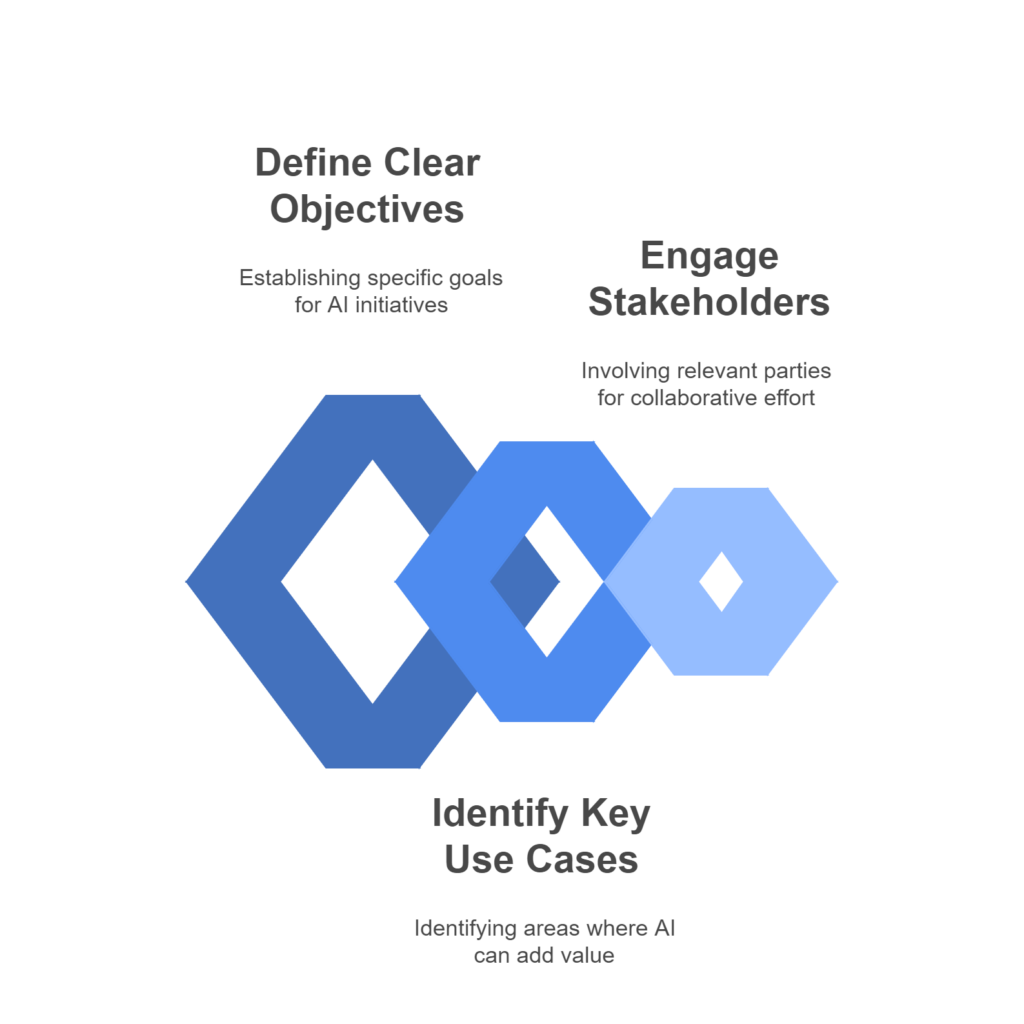
According to Gartner, enterprises with a formal AI strategy are more likely to achieve their digital transformation goals efficiently.
2. Implement a Phased AI Integration Approach
AI adoption should be an incremental process to minimize disruption and allow for continuous improvements.
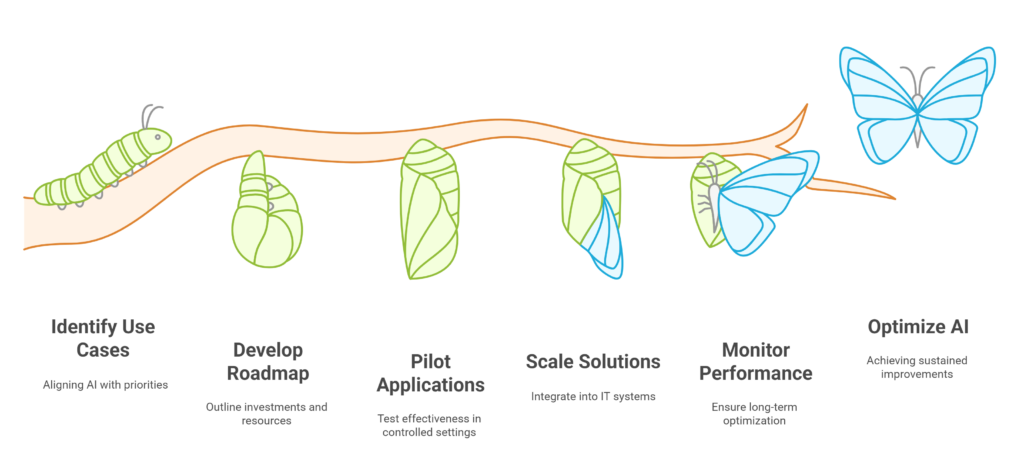
Gartner suggests a five-phase framework for AI integration:
- Identify AI use cases that align with business priorities.
- Develop a strategic roadmap outlining investment planning, resources, and expected outcomes.
- Pilot AI applications in controlled environments to measure effectiveness.
- Scale AI solutions by integrating them into enterprise-wide IT systems.
- Monitor AI performance to ensure long-term optimization and improvements.
This iterative approach allows businesses to assess AI’s impact, make necessary adjustments, and scale its use strategically.
3. Establish a Robust Data Architecture
AI is only as effective as the data it processes. Therefore, a robust data architecture is essential for successful AI integration.
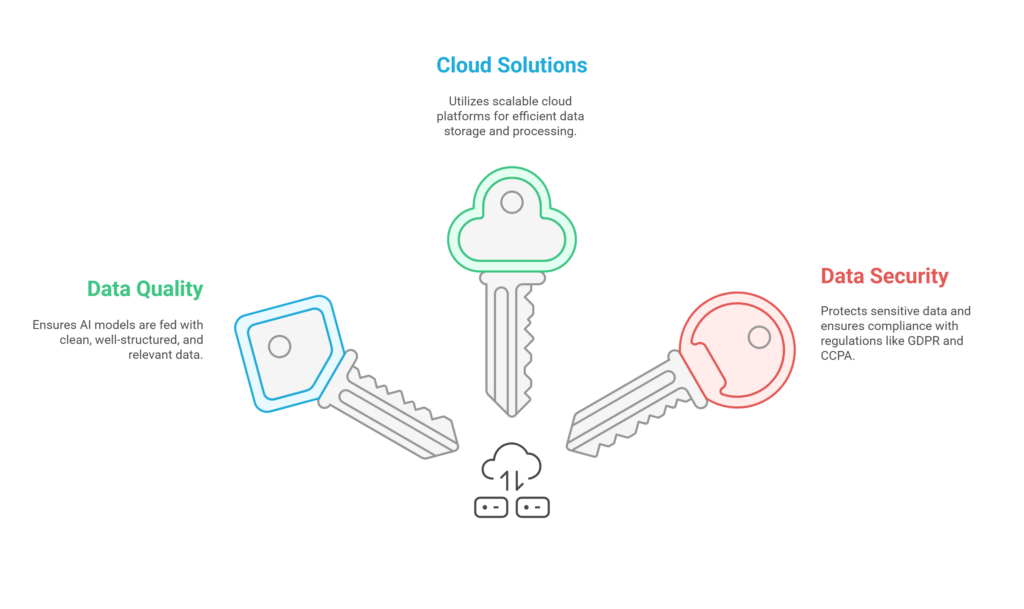
- Ensure High-Quality Data: AI models require clean, well-structured, and relevant data. Implementing data governance practices enhances AI accuracy.
- Utilize Scalable Cloud Solutions: Many enterprises uses cloud-based AI platforms to store and process large data sets efficiently.
- Enhance Data Security and Compliance: Adopting AI necessitates strict compliance with regulations like GDPR and CCPA to protect sensitive data.
A study by MIT Sloan Management Review highlights that AI-driven enterprises with strong data governance outperform competitors by 20% in operational efficiency.
4. Foster a Culture of AI-Driven Innovation
Successful AI adoption requires more than just technical integration—it demands a cultural shift within the organization.
- Upskill Employees: Invest in AI literacy programs to equip employees with the necessary skills.
- Encourage Cross-Functional Collaboration: AI should not remain within IT; departments such as marketing, HR, and finance should also leverage AI-driven insights.
- Leverage AI for Decision-Making: Use AI-driven analytics to support strategic business decisions, rather than relying on traditional intuition-based methods.
Overcoming Challenges in AI Integration
1. Bridging the AI Talent Gap
One of the biggest challenges in AI adoption is the lack of skilled professionals. Organizations must focus on:
- Internal AI Training Programs to upskill existing employees.
- Hiring AI Specialists to lead AI transformation initiatives.
- Utilizing No-Code/Low-Code AI Solutions to democratize AI use across business functions.
2. Managing AI Risks and Ethical Considerations
AI introduces potential risks, including bias in decision-making and regulatory compliance challenges. To mitigate these risks:
- Ensure Transparency and Explainability: AI decisions should be auditable and understandable.
- Adopt Responsible AI Practices: Implement ethical AI guidelines to prevent biases and ensure fairness.
- Monitor AI Performance Continuously: AI models must be regularly assessed to prevent drift and maintain reliability.
Future Trends in AI and Enterprise Architecture
As AI technology continues to evolve, its role in enterprise architecture will expand. Key emerging trends include:
- Composable AI: AI-driven modular architectures allowing businesses to rapidly deploy intelligent systems.
- AI-Enhanced Cybersecurity: AI is increasingly being used to detect and mitigate cyber threats in real-time.
- AI for Enterprise Automation: AI-driven automation tools will streamline business processes, reducing operational costs.
Enterprises that prioritize AI-driven automation will gain a significant competitive advantage in the coming years.
Integrating AI into Enterprise Architecture is a transformative journey that requires strategic planning, robust data management, and a culture of innovation. By adopting a phased approach, ensuring AI governance, and continuously refining AI models, enterprises can successfully leverage AI to enhance efficiency and drive business growth.
With AI becoming a key pillar of digital transformation, businesses that take a proactive approach to AI integration will be better positioned for long-term success.