Enterprise architecture (EA) plays a critical role in the structuring of an organization’s IT and business processes to ensure both efficiency and alignment with long-term goals. But when driven by data, EA becomes even more potent in enabling well-informed strategic decision making. In this post, we’ll explore how Data-Driven Enterprise Architecture (DDEA) optimizes business operations, strengthens business intelligence, and informs data analytics for decision-making with precision.
- Introduction
- The Fundamentals of Data-Driven Enterprise Architecture
- Why Data-Driven Enterprise Architecture is Crucial for Strategic Decision Making
- Case Studies: Companies Leveraging Data-Driven Enterprise Architecture (DDEA) for Strategic Gains
- How Data-Driven EA Supports Long-Term Strategy
- Best Practices for Implementing Data-Driven Enterprise Architecture
- Aligning Data-Driven EA with Your Strategic Decision-Making Process
- Conclusion: The Promise of Data-Driven Enterprise Architecture (DDEA)
Introduction
In its simplest form, Enterprise Architecture (EA) is the blueprint for aligning an organization’s IT infrastructure with its business objectives. Traditional EA helps organizations maintain operational stability by defining which tools, technologies, and processes should be employed. However, there’s a major drawback—traditional EA can be slow to adapt to rapidly changing market conditions.
Enter Data-Driven Enterprise Architecture (DDEA). DDEA amplifies the core principles of traditional EA by infusing it with real-time data, enabling businesses to not only manage their current operations effectively but also to pivot strategically with greater agility. Where traditional EA relies on broad frameworks and best practices, DDEA brings precision. It connects layers of an organization—from customer interactions to backend systems—through a continuous flow of data that informs decision making at every level.
Our thesis is clear: by integrating data-driven methodologies into enterprise architecture, organizations can better navigate a complex, ever-changing landscape. In turn, strategic decisions are made not just based on historical trends but also by leveraging real-time insights—leading to better alignment with market opportunities, risk mitigation, and overall business growth.

The Fundamentals of Data-Driven Enterprise Architecture
What is Data-Driven Enterprise Architecture?
Data-Driven Enterprise Architecture (DDEA) is an evolution of traditional enterprise architecture practices. At its core, DDEA integrates real-time data, continuous analytics, and feedback mechanisms to ensure that the structure underpinning an organization’s IT infrastructure remains adaptable and responsive. Unlike traditional models, which may rely heavily on static processes and historical trends, data-driven EA is built on the premise of fluidity—adapting as new information and market conditions emerge. It establishes a dynamic framework designed not only to align IT with business goals but to actively respond to the business environment as it changes.
Where older models of enterprise architecture take a “set it and forget it” approach, DDEA is anchored in the idea of “evolution through data.” This means regular adjustments, recalibrations, and informed decision-making all stem from live insights rather than outdated metrics.

Connecting Data to Business Goals
One of the main advantages of Data-Driven Enterprise Architecture is how it connects an organization’s data with its strategic objectives. In essence, DDEA serves as an intelligent middle layer between the raw data being generated across an enterprise and the high-level business outcomes that stakeholders are trying to achieve. From optimizing supply chains to improving customer experiences, the architecture continuously evaluates whether current technical frameworks are adequately driving business objectives and indicates where recalibrations are needed.
For example, a retail company might be collecting customer data from both in-store purchases and online platforms. DDEA doesn’t just warehouse this information; it translates insights into actionable objectives, such as improving inventory management to match customer demand patterns in real-time. This ensures that operations remain in lockstep with changing business objectives, eliminating costly misalignments.
Key Components of Data-Driven EA
To truly harness the potential of DDEA, certain foundational elements need to be in place. These core building blocks facilitate both the data flow and its strategic use within the enterprise.

- Data Collection Frameworks
The backbone of DDEA is its ability to intake raw data continuously. This doesn’t just mean gathering the data but doing so strategically—integrating diverse sources such as IoT devices, application logs, customer feedback mechanisms, and market analytics into cohesive streams. By building strong data collection frameworks, organizations ensure that they have consistent visibility into every facet of operations. - Analytical Tools
Once data is captured, analytics come into play. High-quality analytical tools transform raw information into digestible insights, ranging from trend forecasts to diagnostic analysis. Tools like AI-driven algorithms or machine-learning platforms augment enterprise architecture by providing advanced decision-support, helping teams predict patterns, uncover inefficiencies, or even automate decision pathways. - Integration with Existing Business Processes
Creating an architecture driven by data requires seamless integration with existing operational systems, ensuring the data flows naturally where it’s most needed. Whether it’s integrating with financial software, CRM platforms, or supply chain management tools, the architecture needs to interact harmoniously with the tools already in place. Integration ensures that the insights generated from analytics have a direct path into the action zone—meaning insights quickly transform into decisions and outcomes.
In short, Data-Driven Enterprise Architecture doesn’t stop at collecting or analyzing data. It builds a feedback-rich ecosystem where continuous learning informs better decisions, realigns technical processes with business goals, and allows enterprises to remain agile and proactive.
Why Data-Driven Enterprise Architecture is Crucial for Strategic Decision Making
In today’s competitive landscape, the capability to make quick, informed decisions can be the difference between thriving and merely surviving. Typical enterprise architectures, while effective at stabilizing internal operations, often struggle to deliver the agility modern businesses need. Here’s where Data-Driven Enterprise Architecture (DDEA) steps in. By integrating real-time data and predictive analytics into the structure of an organization, decision-makers can move from reactive to proactive strategies. Let’s dig into why this shift is essential:
1. Enhanced Business Intelligence and Insights
Enterprise-wide Data Consolidation
One of the most significant barriers to informed decision-making is data silos. Different departments often operate with their own isolated datasets, resulting in inefficiencies and a disjointed view of the business. A data-driven enterprise architecture breaks down these silos, consolidating data from various sources into a unified framework. When companies have seamless access to this integrated data, it becomes far easier to gain 360-degree insights — from understanding customer behavior trends to monitoring operational bottlenecks.
Timely and Meaningful Insights
In a fast-moving business environment, actionable data isn’t just useful; it’s crucial. By implementing DDEA, organizations can use real-time analytics to not only understand the “what” of past events but also reliably forecast future scenarios. For example, knowing sales trends from the previous quarter is the baseline. What really drives strategic value is being able to predict next quarter’s deviations and act on them in advance. Consider the case of a retail company leveraging predictive analytics via DDEA to anticipate a shift in consumer demand and adjust its distribution strategy days before competitors catch on. That’s strategic intelligence in action.
2. Real-Time Data Analytics
Data as a Source of Truth
Of course, your insights are only as good as the data they come from. Traditional enterprise architecture often bases decisions on static or, at best, slow-to-update datasets, which leads to skewed or outdated takes on current market conditions. In contrast, DDEA provides a live feed of operational and market data, ensuring that decisions are grounded in the most up-to-date information available. The result? More accurate, consistent decision-making, with fewer surprises and minimized guesswork.
Diagnostics and Predictive Power
Take the example of a global retail company that switched to DDEA. Previously, they were experiencing frequent disruptions in their supply chain, due in large part to their outdated, piecemeal approach to data handling. By centralizing their data sources and incorporating real-time diagnostics, they could now predict timing and location of potential bottlenecks with remarkable precision — leading to an 18% efficiency improvement in their overall resource allocation.
Faster Adaptation to Market Changes
In industries where the landscape shifts overnight, speed is a strategic necessity. A prime example is the financial sector, where conditions like interest rates or commodity prices can alter consumer behavior in real-time. A financial institution that embraces DDEA can instantly translate these shifts into actionable insights. One particular financial firm achieved a 25% reduction in risk exposure, thanks to the agility provided by a constantly evolving, real-time data ecosystem. They could see impending changes in the market with fine-tuned accuracy, empowering them to swiftly reallocate resources while competitors lagged behind.
DDEA represents a tipping point in how businesses approach long-term strategy. Instead of making decisions on historical trends or gut instinct, you’re operating on a live wire of actionable data that is both accurate and predictive—laying the foundation for smarter, faster strategic choices.
Case Studies: Companies Leveraging Data-Driven Enterprise Architecture (DDEA) for Strategic Gains
Case Study 1: Manufacturing Industry
- Scenario:
A large-scale manufacturing company was facing issues with disconnected systems and delayed data. The inability to predict equipment failures led to frequent lengthy downtimes, worsening production inefficiencies and resulting in missed delivery targets. - Solution:
Implementing a data-driven enterprise architecture (DDEA) that integrated IoT data from machinery with financial and operational systems created a real-time unified data stream. This enabled visibility into machine performance metrics, allowing for early detection of wear and tear. - Outcome:
The integrated data stream improved production forecasting, reducing downtime by 15%. Furthermore, the company’s product development timelines became faster due to streamlined processes, leading to an overall production efficiency increase of 12% within two years.
Case Study 2: Financial Services
- Scenario:
A financial services institution was struggling with fragmented risk management across its various business units. This led to an inconsistent integration of trading, compliance, and portfolio data, exposing the institution to significant market risks, especially during times of high volatility. - Solution:
By adopting a data-driven enterprise architecture, the institution centralized its risk analytics to create a unified, real-time data platform. The new system integrated trading data, market insights, and regulatory updates. Machine learning algorithms further enhanced its predictive capabilities, allowing dynamic adjustment of investment strategies. - Outcome:
The institution reduced its overall market risk exposure by 25% within a year. Additionally, increased visibility into risk triggers and more agile decision-making led to a profitability boost of nearly 12%. The company became more resilient in the face of an unpredictable financial environment.
By leveraging the power of a data-driven enterprise architecture, both companies were able to achieve significant operational improvements, leading to lower risks, greater efficiency, and ultimately, stronger bottom-line results.
How Data-Driven EA Supports Long-Term Strategy
1. Adaptability at Scale
One of the most significant advantages of Data-Driven Enterprise Architecture (DDEA) is its inherent flexibility and ability to scale with the business. In environments where customer demands, market conditions, and technological advancements shift unpredictably, traditional architecture is often too rigid to support rapid adaptation. DDEA, however, enables systems to evolve continually by integrating live data feedback and analytics directly into operational and strategic frameworks.
This adaptability benefits businesses in multiple ways. For instance, companies that have embraced data-centric approaches to their enterprise architecture report up to a 22% improvement in handling market pivots, responding to competitor moves, or capitalizing on emerging trends. Data serves as the compass, allowing the architecture to adjust in real-time and ensure alignment with business goals while also supporting innovation. The key takeaway? If a company’s infrastructure can change as fast as the market demands, it’s better positioned to thrive.
2. Driving Digital Transformation
Another critical factor where DDEA shines is in powering digital transformation initiatives. Digital strategies often fail because they are not effectively backed by flexible, data-driven infrastructures that can support rapid technological integration and orchestration. Here, DDEA acts as the backbone for any major digital shift.
By feeding real-time analytics into enterprise systems, businesses can fast-track initiatives like process automation, customer experience personalization, and agile product development with precision. Digital transformation becomes less about massive, single-step changes and more about incremental growth, continuously adapting to new digital tools and consumer demands.
More than just talk, early adopters are already demonstrating the efficiencies this brings. For example, organizations leveraging real-time insights at the core of their EA have managed to reduce time-to-deploy on new digital tools by 30%, giving them a competitive edge in reacting swiftly to customer needs and market pressures. Ultimately, a DDEA isn’t just about keeping up; it becomes the engine driving tomorrow’s innovations.
Best Practices for Implementing Data-Driven Enterprise Architecture
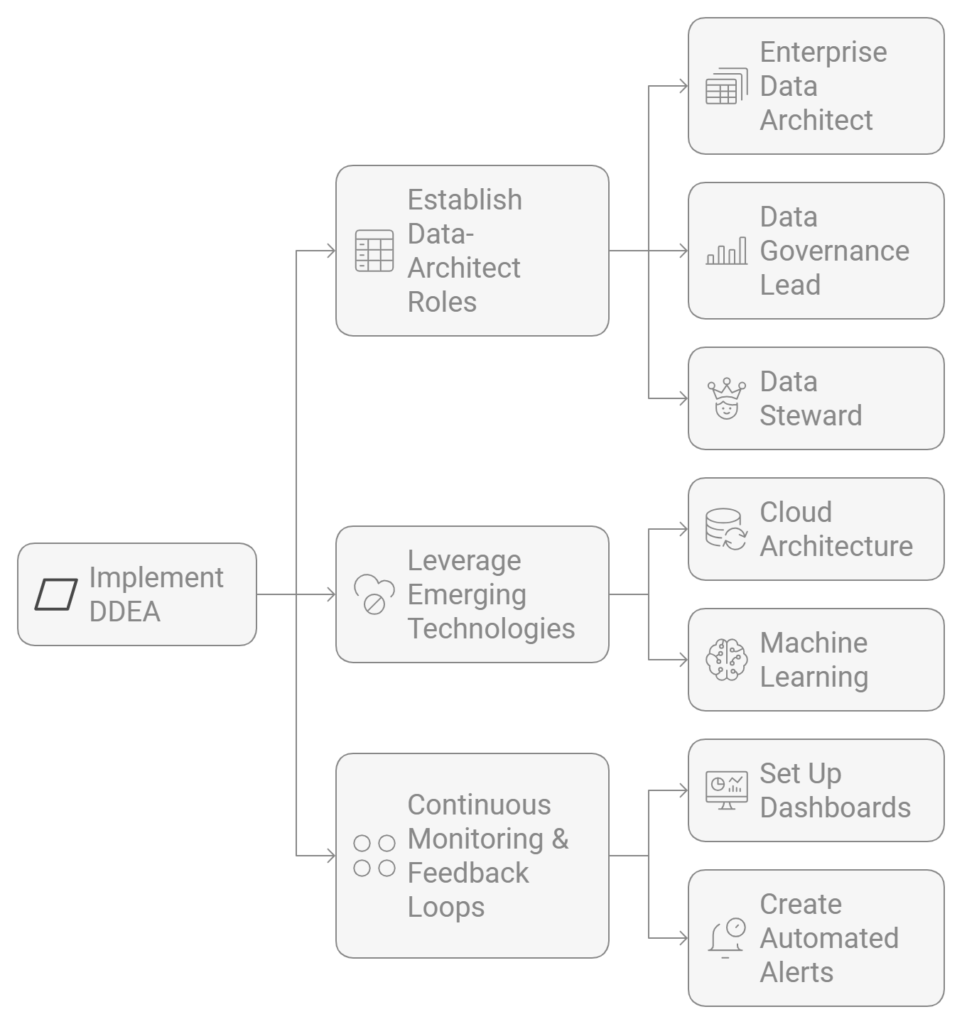
1. Establish Data-Architect Roles and Governance Models
One of the foundational steps in implementing a Data-Driven Enterprise Architecture (DDEA) is clearly defining key roles within your data governance ecosystem. The Enterprise Data Architect, Data Governance Lead, and Data Steward roles are pivotal in ensuring that data is not just available but structured, secured, and in compliance with evolving regulations.
- The Enterprise Data Architect is responsible for designing the overarching data structure to align with business goals. They define how data flows will integrate with the existing architecture.
- The Data Governance Lead establishes guidelines for data quality, security, and ownership to ensure each department knows how to handle the information.
Alongside these roles, it’s essential to create a data governance structure that ensures accountability while facilitating efficient data handling. Without proper governance, your data can quickly become an unmanageable, compliance-risking burden rather than a strategic asset.
2. Leverage Emerging Technologies
Once you have your governance in place, make sure you’re taking full advantage of today’s cutting-edge technologies that can turbocharge your data architecture.
- Cloud Architecture & Big Data Integration: Cloud platforms such as Google Cloud, AWS, and Azure provide the elasticity and localized infrastructure needed for scaling operations, making them an ideal fit for companies looking to integrate large-scale data analytics into their EA. Not only do these platforms support large workloads, but they also come with built-in analytical features — letting your DDEA move smoothly with real-time data at manageable costs.
- Machine Learning in Decision-Making: Machine learning (ML) is more than just predictive analytics sprinkled in your system. When embedded within your architecture, ML algorithms allow you to anticipate everything from operational bottlenecks to financial forecasting with increasing accuracy. Companies like UPS, for instance, have integrated ML within their EA to predict truck maintenance and route optimizations, blurring the lines between reactive and proactive decision-making.
3. Continuous Monitoring and Feedback Loops
Real-time data flows can generate overwhelming amounts of information. Therefore, an effective DDEA needs to implement continuous monitoring mechanisms, harnessing real-time analytics without drowning in the data.
Set up dashboards that track the most valuable KPIs tied directly to your strategic goals. This is where the feedback loop becomes critical. By continuously analyzing the output of these metrics, organizations can adapt their architecture in real time, making course corrections when trends show inconsistencies or inefficiencies.
Create automated alerts that feed directly into the operational loop, providing stakeholders with actionable insights as conditions change. Let your architecture evolve every day — not just during quarterly reviews — to match the ever-changing market landscape.
Aligning Data-Driven EA with Your Strategic Decision-Making Process
To fully harness the power of Data-Driven Enterprise Architecture (DDEA), alignment with your existing strategic decision-making processes is essential. It’s not just about implementing the right technology—it’s about fostering a culture of data-first thinking and integrating this directly into top-level strategies. Here’s how to build that bridge between your data-driven architecture and effective, actionable decisions at all levels of the organization.
1. Embed DDEA in C-Suite Strategic Meetings
For DDEA to make the impact it’s capable of, it needs to be a foundational piece of all high-level policy and planning discussions. Executives should routinely ask for data-backed insights during strategy sessions, using enterprise-wide current analytics to shape outcomes. Action Items:
- Integrate dashboards and real-time data from your enterprise architecture into all executive meetings, enabling a data-led discussion on operational performance, resource allocation, and strategic pivots.
- Make predictive analytics a standing item in C-suite agendas—whether it’s forecasting market dynamics, operational risks, or financial performance metrics. This keeps the focus on forward-thinking decisions, minimizing reactionary responses to problems.
2. Adapt DDEA to Agile Road Maps
Just as DDEA thrives on continuous data flows, business strategy benefits hugely from agility at both the strategic and operational levels. Agile road maps, which prioritize flexibility and quick iteration, are an ideal partner for data-driven decision-making.
- For Project Teams: Incorporate a data feedback loop into sprint planning. Require data-analysis checkpoints so that teams can adapt their cadence based on real-world insights rather than static assumptions.
- For Managers and Strategists: Use data to inform the prioritization of work items in agile road maps or quarterly OKRs (Objectives and Key Results). Rather than relying on outdated intuition, ensure the highest-value initiatives are based on today’s data.
3. Establish Departmental Data Pipelines
The application of data-driven decisions shouldn’t stop at the executive level. More valuable strategic alignment happens when every part of the company can plug into enterprise intelligence.
- Cross-department Data Flow: Foster cross-functional teams that work directly on interpreting data generated by EA. Whether it’s finance, operations, or marketing, every department should have access to actionable insights specific to its function. This provides an organization-wide commitment to making informed, data-first decisions at every level.
- Integrate into KPI Reviews: Align departmental goals and KPIs with data-driven outputs from your DDEA structure. Regularly cycle through key performance metrics as informed by real-time data. The result: each department not only aligns with strategic objectives but can quickly recalibrate when new information demands a shift.
4. Build a Data-Driven Decision Culture Organization-Wide
Perhaps the hardest part of aligning DDEA with decision-making is answering the behavioral question: can your entire organization build data into its DNA? Data-driven culture isn’t just about setting rules; it’s about conditional procedures empowering decision-making across all roles—not just the IT or analytics teams.
- Education on Tools & Methods: Invest in data literacy at all levels of the company. Equip leaders and managers with actionable knowledge of the analytical tools at their disposal (dashboards, AI models, predictive tools) and ensure they are comfortable interpreting the results.
- Encourage Questions: Create an open environment where “Is there data to support this?” becomes a frequent consideration in meetings from entry-level discussions all the way to board meetings. Promote a culture of evidence-based challenge when decisions appear instinct-driven rather than fact-driven.
By embedding DDEA in strategic activities at every level (from executive boards down to agile teams and line managers), you transform your enterprise architecture from a supportive IT framework into a trusted core for real-time, informed decision-making.
Conclusion: The Promise of Data-Driven Enterprise Architecture (DDEA)
Data-Driven Enterprise Architecture (DDEA) isn’t just another IT trend; it’s becoming the backbone of intelligent, future-proof strategic decision-making. By integrating dynamic, real-time data into their architecture, enterprises are moving from reacting after changes happen to anticipating them—responding before the full effects take shape.
Key Takeaways
- Centralized Data: DDEA centralizes data from disparate systems, eliminating guesswork and increasing data reliability.
- Enhanced Business Intelligence: Through clearer insights, business intelligence is elevated, providing leaders with actionable data to drive decisions.
- Agility & Adaptability: Enterprises gain greater flexibility to respond to evolving market conditions, ensuring they stay competitive.
These capabilities do more than just streamline operations—they serve as competitive differentiators, delivering the kind of foresight that was unattainable just a few years ago.
Continuous Improvement is Essential
However, a successful DDEA implementation requires consistent effort:
- Ongoing Evolution: The technological landscape is constantly shifting, and so too must your systems.
- Data Validation: Active data validation is critical to ensure that your strategic insights are grounded in accuracy.
An effective DDEA strategy is an ongoing journey—one that consistently aligns technology with your business goals, ensuring both short-term results and long-term success.
Time to Act
Markets are growing more complex, and opportunities more fleeting. Now is the time to harness the full potential of data in your enterprise architecture. Doing so could unlock new layers of strategic opportunity and maximize the benefits of foresight in today’s fast-paced environment.
The next move is yours—will you make it data-driven?